I cut my teeth on the General Motors (GM) plant floor almost 25 years ago, working in both the body shop, which was ruled by robots, and the general assembly area, which was dominated by people. I learned very quickly that data from robots was easily accessed over the ethernet, but data pertaining to humans had to be generated manually by industrial engineers wielding stopwatches, performing the same time studies that Frederick Taylor introduced around the time of the Model T. The contrast was stark even then: the modern industrial age bumping up against a data-collection method that was already around 75 years old.
A quarter century later, I still walk assembly areas and see young engineers performing time studies. The tools have changed — they’re more likely to use an iPhone instead of a Casio — but the activity remains identical, which upsets me. Time studies (and motion studies) were pioneering techniques. They made American manufacturing the best in the world. But they’re also deeply flawed. In the age of the industrial internet of things (IIoT), time studies can’t keep up.
Focus on Time to Market and Productivity
There’s no doubt American manufacturing is on the upswing, expanding at the fastest pace since 2004. In 2017, 171,000 manufacturing jobs came back to the United States as a result of reshoring and foreign direct investment. Driving this resurgence, perhaps chief among many reasons, is market access.
“Reshoring is all about time to market,” says Ben Smith, senior advisor at consulting firm AT Kearney. “It’s not just a matter of finding an old factory in Pittsburgh and turning the lights back on. Modern manufacturers may very well prefer 15 small factories distributed across the country for better market access, which means the factory managers shift their focus from costs to productivity.”
The conventional wisdom is that productivity will be increased by the IIoT. According to Accenture, the IIoT is arguably the biggest driver of productivity and growth in the next decade. GE Digital believes the IIoT will be responsible for performance gains that value approximately $8.6 trillion on an annual basis.
One problem is that most of the value a factory creates doesn’t come from its machines but from people. A Boston Consulting Group study found that people still perform up to 90 percent of tasks on the factory floor. So, you can optimize your machines until the cows come home, but you’re only optimizing 10 percent of what’s happening in your plant.
Time to Market Bumps Against Time to Data
“On any given production line, only a small percentage of tasks are performed by machines,” says Peter Marcotullio, vice president of commercial research and development at SRI International. “If you’re instrumenting machines, you’re only capturing a fraction of the process.”
I understand the attraction of the IIoT. Look at the size of the dataset. In the time it would take you to conduct a time study or fill out a standardized work chart, you may have 50 gigabytes of machine data for your predictive analytics engine. It’s simple to calculate overall equipment effectiveness (OEE) to three decimal places. If time to market is of the essence, then time to data must count for a lot.
But there’s no straight line between optimizing equipment and improving overall plant productivity. When you look at the contribution of machines as a percentage of the value creation, you see that the IIoT misses the big picture. Some of the most critical decisions a plant manager makes — everything from daily staffing and output projections to job costing and quoting — requires data from people. Data from people drives the top and bottom line, which is why I worry so much when I see people running time-and-motion studies even today.
An Incomplete and Unreliable Dataset
There are two problems with time studies: the size of the dataset and the trust it deserves. First, time studies are incomplete. An engineer gets a pat on the back if he or she returns from the line with 10 samples per station. But 10 samples aren’t significant enough to account for operator variability. Does it matter if the samples are taken on a Monday morning or a Friday afternoon? On payday or the day after? There isn’t enough data to establish correlations and causality.
This leads to the second issue: data reliability. Werner Heisenberg demonstrated that the very act of observation can perturb an observed system. What’s true for quantum physics is just as true for workstations. Some workers move faster to impress an observer; others slow-walk their work to pad the standard they know they’re creating for themselves. Compounding this difficulty are measurement challenges. For example, an observer must consistently determine when an activity starts and ends.
Time studies result in measurements that are dubious at best. This impacts the conclusions one draws from them. It also hurts the operators as much as it hurts the business, because if time to market requires data, and data can’t easily come from people, then manufacturers over-index on machines. People lose jobs.
What’s Missing from the Modern Plant?
There are many ways to augment your dataset on people: regular Gemba walks, instrumented light curtains and other poka-yoke systems that collect data, and countless physical hooks into the manufacturing execution system (MES) to compute cycle time and infer productivity. But this data doesn’t scale. Data on human assembly activities are effectively invisible to analytics.
“When a manufacturer wants to resolve a production issue, they gather the workers and the managers to brainstorm ideas,” says Anik Bose, general partner for Benhamou Global Ventures. “If they want to improve asset utilization, they bring in lean experts for time-and-motion studies or rely on twice-yearly visits by engineers. For new product introductions, they shut down the line to run experiments. It’s all manual and ad hoc because they have no alternative. They have no real-time data that enables any other type of approach.”
If plants can barely measure human productivity, how are they going to improve it? And if they can’t improve human productivity, what happens to the human operator? I think back to Marshall Goldsmith’s famous quote: “What got you here will not get you there.” The time study got us here, and for that I thank Mr. Taylor. But when I look to “there,” which I see as accelerating time to market from increasing (human) labor productivity, I’m convinced that the time study’s time has come.
About the Author
Dr. Prasad Akella led the team that built the world’s first collaborative robots at GM. He is the CEO of Drishti, a new company that deploys artificial intelligence to collaborate with and enhance humans on the factory floor.
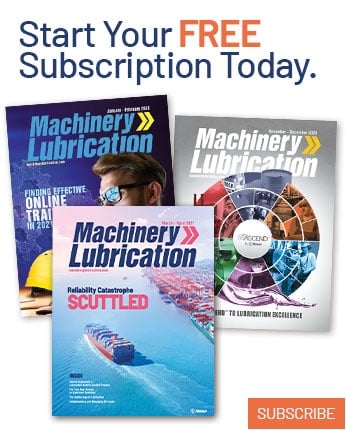