For years, maintenance has been treated as a dirty, boring and ad hoc job. It’s critical for productivity but isn’t recognized as a key component of revenue generation. The simple question is often, “Why do we need to maintain things regularly?” The answer is, “To keep them as reliable as possible.” But the real question is, “How much change or degradation has occurred since the last round of maintenance?” The answer is, “I don’t know.”
The challenge for reliability is dealing with data from the past. Failure is modeled, analyzed and, to some extent, predicted. Unfortunately, the prediction doesn’t take into account users or working environment-related constraints, and often the results aren’t that useful.
Condition-based maintenance (CBM) deals with online data. Machine conditions are constantly monitored and their signatures evaluated. However, this is done at the machine level – one machine at a time. It’s a “fail-and-fix approach.” Troubleshooting is the primary purpose.
Today, CBM focuses on sensors and communications. All products and machines are networked by some means. It’s hard to know, though, what to do with all this data. We must turn data into information by using computational tools to process data locally.
The maintenance world of tomorrow is an information world for feature-based monitoring. Information should represent a trend, not just a status. It should offer priorities, not just show “how much.” If we do that, then our productivity can be focused on asset-level utilization, not just production rates.
With the advent of modern computing and communication technologies, more products and machines are embedded with sensors and connected through tethered and tether-free networks. With the seamlessly integrated network systems in today’s global business environment, machines and factories are networked and information and decisions are synchronized, so any downtime in the system can jeopardize the productivity of the entire enterprise.
On the other hand, constraints on security and communication bandwidth limitations require re-engineering to minimize or mitigate data exposure risks.
The solution is intelligent maintenance systems. IMS predict and forecast equipment performance, so “near-zero breakdown” status is achievable. There are two reasons for failure: equipment performance and human error. Near-zero downtime focuses on predictive techniques to minimize failures. It focuses on features of machine performance.
Data comes from two sources: sensors (mounted on the machine to gather the feature information) and the entire enterprise system (including quality data, past history and trending). By correlating data from these sources (current and historical), you can predict future performance. The goal is to predict product/machine health in the same way that weather is forecast.
We really don’t care about how precise the temperature prediction is. We care about the trend – cold to hot or clear to rainy. Forecasting gives us priorities and a backup plan.
Today, machine field services depend on sensor-driven management systems that provide alerts, alarms and indicators. The moment the alarm sounds, it’s already too late to prevent the failure. Most factory downtime is caused by these unexpected situations.
There is no alert provided that looks at degradation over time. If we can monitor degradation, then we can forecast upcoming situations and perform predictive reliability tasks when necessary (not too early or too late). Using these techniques, maintenance can be scheduled prior to failure.
My long-term view on intelligent maintenance is that we can use any means – including embedded (software) and remote technologies – to monitor equipment performance. Then, if degradation starts to occur, there is enough time to trigger service before failure.
A machine can self-assess its health and trigger its own service request as needed. If this model works, then we will have a product that can manage its own service performance and its own warranty-based contracts. It also can alert us on ways to keep it running in a high-performance manner.
Industry is so focused on the bottom line, and the cost of downtime largely impacts profitability. Yet downtime rates are not the only cost concerns. If equipment starts to degrade, you may start generating parts with unacceptable quality and not know it for a long time.
Eventually, machine degradation will seriously impact throughput and quality. This is especially important for manufacturers that outsource operations to countries like China. These firms need to remotely monitor the quality of products before they ship.
World-class companies already have taken a game-changing approach of predictive reliability, implementing a new service business model to transform maintenance systems into smart service and asset management solutions.
They reduce downtime and provide the ability to look ahead at the quality of products before they ship by closely watching equipment performance and machine degradation. Rather than reactive maintenance – “fail-and-fix” – companies move to “predict-and-prevent” maintenance.
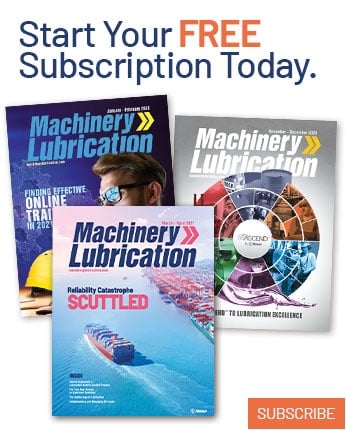