Introduction: Generative AI and Its Role in Reliability-Centered Maintenance
Reliability-centered maintenance management has undergone significant evolution over the past several decades. Currently, the most widely used approach is condition-based maintenance, which leverages real-time sensor data and monitoring systems to trigger maintenance only when specific operational thresholds are exceeded, improving efficiency. In the last few years, industry leaders have adopted a new approach based on prescriptive maintenance, which combines machine learning with historical and real-time data to predict failures and recommend optimized maintenance actions. Now, Generative AI (GenAI) represents the next major shift in reliability management, offering the ability to process vast amounts of structured and unstructured data and generate intelligent, adaptive solutions.
Generative AI differs from traditional machine learning models. It not only finds patterns in data; it brings together inputs from diverse sources: sensors, logs, manuals, past maintenance work, even notes or conversations from engineers on the floor. Where traditional models might flag correlations, generative systems can simulate options, explore outcomes, and suggest better paths forward based on current conditions. For example, a GenAI-powered system can analyze machine vibration data, correlate it with historical failure patterns, and not only predict a potential bearing failure but also suggest an optimized replacement schedule based on operational load and supplier lead times.
Agentic AI, a more advanced form of generative technology, pushes this even further. Designed to act autonomously within defined rules, it doesn't just analyze; it decides and follows through. In reliability work, this means systems can plan maintenance through interaction with CMMS and ERP systems, adjust equipment operations, or even fix problems before anyone notices.
When repetitive jobs are handled automatically, reliability engineers can step back and focus on high-value activities like improving system design and optimizing asset performance. This shift from fixing problems after they happen to preventing them before they start fundamentally transforms reliability practices, leading to more uptime, less waste, and longer-lasting assets.
The Architecture of Agentic AI for Reliability
Modern reliability programs rely heavily on CMMS (Computerized Maintenance Management Systems) and RCM (Reliability-Centered Maintenance) frameworks. Agentic AI architecture builds upon these foundations by creating an intelligent system that autonomously manages reliability operations while integrating seamlessly with existing platforms.
The architecture consists of four integrated layers that work together to transform maintenance practices from reactive to predictive and autonomous:
- Data Acquisition Layer: Acts as a bridge between existing systems and new AI capabilities. It pulls information from CMMS work orders, sensor data, third-party external datasets, and maintenance records while categorizing them according to RCM principles like failure modes and criticality rankings.
- Knowledge Processing Layer: Transforms data into actionable intelligence, applying RCM logic to contextualize its analysis. For example, it considers both real-time readings and the asset's criticality rating and documented failure modes when evaluating equipment data. It cross-references historical maintenance records, manufacturer specifications, and industry best practices to determine effective responses.
- Decision Intelligence Layer: Operates within RCM-established boundaries while enhancing decision-making with AI. It automatically updates CMMS maintenance schedules, adjusts preventive maintenance frequencies, and optimizes resource allocation based on real-time conditions, ensuring alignment with reliability strategies.
- Action Execution Layer: Implements decisions through existing systems, generating CMMS work orders, updating asset records, and maintaining audit trails. Built-in feedback mechanisms ensure the system learns from maintenance outcomes, creating a self-improving cycle. Robust security measures, validation checks, and escalation paths are included to prevent AI decisions from overriding critical safety parameters.
This comprehensive framework ensures that Agentic AI enhances, rather than replaces, established maintenance practices while maintaining the strategic importance of human expertise and oversight.
How Agentic AI Transforms Reliability Operations
Agentic AI is transforming reliability operations by enabling autonomous, data-driven decision-making at scale. The focus is not just on predicting failures but also on recommending and executing corrective actions in real time.
For example, an Agentic AI system monitoring a steam turbine can detect a rise in vibration levels, cross-reference it with historical failure data, and predict a bearing failure. The AI model can then recommend adjusting lubrication schedules and operating loads to prevent damage. If the issue persists, the AI can automatically generate a work order, notify the maintenance team, update CMMS systems, and even reorder replacement parts through an integrated ERP system.
The key advantage of Agentic AI lies in its ability to simulate multiple scenarios, weigh operational trade-offs, and select the most effective course of action autonomously. It also enhances root cause analysis and failure diagnosis by correlating diverse data sources—sensor logs, technician notes, and technical manuals—to generate comprehensive diagnostic reports. By automating these processes, Agentic AI increases operational uptime, reduces maintenance costs, and extends asset lifespan.
Risks and Mitigation Strategies
Agentic AI brings important challenges, particularly regarding explainability, traceability, and responsible oversight. It is essential that decisions made by these systems are understandable, especially in reliability-focused environments.
To improve transparency, organizations can blend traditional rule-based logic with adaptive models, making the system's reasoning easier to follow. Detailed reports outlining data sources, confidence levels, and decision trade-offs can further support understanding. Simulated environments provide teams with the opportunity to explore and test system behavior before full deployment.
Maintaining a clear record of system behavior is crucial. Documenting inputs, outputs, and key decisions ensures a traceable trail for review and compliance. Monitoring version history and system performance metrics like mean time between failures allows organizations to evaluate system effectiveness over time.
Even as systems become more autonomous, human oversight remains vital. Built-in safeguards should flag unusual or high-stakes situations for operator review. Requiring approval for sensitive decisions and incorporating ongoing user feedback ensures continuous system improvement.
When clarity, traceability, and oversight are embedded in system design, organizations can safely capture the benefits of automation while maintaining accountability and safety.
The Future of Autonomous Reliability Management: Conclusion & Takeaways
The future of reliability management is moving toward intelligent, autonomous systems that minimize the need for human intervention while boosting accuracy, speed, and efficiency. Agentic AI will continue to advance with better machine learning capabilities, deeper contextual understanding, and real-time adaptability.
Tighter integration with CMMS and ERP platforms will allow full automation, from detecting issues to creating work orders, assigning resources, and managing inventory. AI will not just forecast complex failure trends but also suggest corrective steps and operational tweaks to enhance asset performance.
Agentic AI will increasingly handle routine diagnostics and maintenance tasks, freeing reliability teams to focus on higher-value activities like system design, performance optimization, and innovation. Roles will shift from repetitive maintenance tasks toward strategic oversight and innovation.
As AI becomes more capable, organizational success will hinge on balancing automation with human judgment. Ensuring that automated decisions align with regulatory requirements and broader business goals will be essential. The true breakthrough in reliability will come from how effectively companies embed AI, manage its risks, and evolve alongside it, creating a future that is predictive, resilient, and continuously improving.
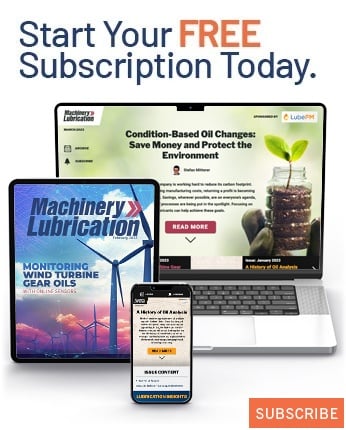