Knowing that we have the ability to act before we encounter difficulties gives us a feeling of security. In a production process, this secure feeling is accompanied by cost savings, customer satisfaction and the ability to face official inspections calmly.
Statistical Process Control (SPC) has been around for a long time. But only in the last several years have many modern companies have begun working with it more actively – not least because of the propagation of comprehensive quality systems, such as ISO, QS9000, Six Sigma and MSA (Measurement System Analysis).
SPC is far more than a control chart or a mere capability index. It is a system that uses process data to describe a prototypical manufacturing process in connection with its environment. The goal of the method is to intervene in the process before tolerance violators occur, and thereby optimize the entire process. The method uses a variety of elements, which in their totality form the SPC module of FreeWeigh.Net. Control limits, CuSum, specification limits, cp and cpk are the elements available in FreeWeigh.Net that allow you to have even better control over the processes being monitored, to document them, and, if needed, to intervene even faster. The following sections describe the individual elements and their benefits in greater detail.
The heart of SPC: normal distribution
Normal distribution as described by C.F. Gauss (1777-1855) with its typical Gaussian distribution curve (also called the bell curve) lies at the heart of the mathematical model used to illustrate Statistical Process Control. Normal distribution is based on the principle of a limitless totality. Since more than 200 samples already allow a good approximation, this relatively simple model is sufficient for describing processes in a suitable way. The distribution generated by the model is described in terms of the mean and the standard deviation. In addition, the difference, minimum value and maximum value, sample size, and tolerance limits and specification limits also play a role. A basic knowledge of these individual terms is needed in order to understand how they work together.
Control limits
In contrast to tolerance limits, which are used for the individual values of a sample series, control limits are used for the mean and the mean variation of a series. These control limits are like guardrails that are narrower than the tolerances for the individual values.
The control limits for the mean value are defined with three parameters: the upper and lower control limits, and the target value for the mean. As soon as the mean (and not an individual value) goes beyond this guardrail, an appropriate distribution can be shown. As a rule, the target value for the mean is slightly higher than the nominal, since in the food industry the mean value for packaged products must equal or exceed the nominal over a defined period (e.g. batch).
No general recommendation can be given for the initial value of any of these three parameters. Although the sample series comprises individual products taken one after the other from the production process, other factors, such as the mean variation of the process and environmental influences, play a key role. This is why we suggest setting the initial values for the upper and lower control limits to approximately 60 to 70 percent of T1.
With the SPC module from FreeWeigh.Net, however, the control limits can also be continuously calculated. This is particularly advisable when a relevant process mean variation (through decisive influential factors that can change over time) should be considered. The time of this recalculation is only practical, however, if a representative set of data was recorded between the calculation times. Moreover, the calculation does not depend on the size of the sample, and is based on the conventional trade factors of A2 and A3 for the mean value, B3 and B4 for the standard deviation, and D3 and D4 for the spread. The sample size used for the calculation can also be defined. The smaller the number selected, the larger the influence of the current measurement value and the faster an alarm can theoretically occur. If a large value is selected, this effect is lessened.
In addition to mean value monitoring, FreeWeigh.Net also allows control of the spread or the mean variation. In most cases, the initial value for monitoring the mean variation is the same or slightly higher than the mean variation of the process. On the other hand, the mean variation of a sampling series can never be zero because this would contradict the theory of normal distribution. With a lower and upper limit for the mean variation, it can be maintained within a defined tolerance. We view the monitoring of the mean variation as a suitable tool for the food industry because a separate report can be configured in the case of a tolerance violation (and its associated large spread).
The monitoring of the spread limits the difference between the maximum and minimum value within a sample. With two limits, this tolerance is also defined in such a way that the lower limits are, more appropriately, not zero. In theory, a series of products will never exactly show the same measuring results – the use of measuring instruments with suitable resolution is taken for granted, however. The upper limits define the maximum difference between the extreme values of a sampling series. This monitoring is especially appropriate for checking for the kind of uniformity required, for example, by the various pharmacopeias.
No matter the basis selected, this function can be used to monitor processes within a narrower tolerance. This gives the user the security of knowing that he or she will receive sufficient warning well before a real tolerance violation, and that he or she can then take appropriate measures.
CuSum: Depiction of the mean values
CuSum is used for an exaggerated depiction of a trend. Based on the deviations of the sample mean values to the target value (often identical to the nominal value), these differences are cumulated over time. To depict this trend, two CuSum curves are computed and shown – an upper curve for positive deviations and a lower curve for negative deviations. As long as the deviations show a positive value, the upper CuSum curve keeps rising, each time by the value that shows the difference between the current sample mean value and the nominal value. If this difference remains positive, this trend line will exceed a limiting value sooner or later. FreeWeigh.Net can be configured to sound an alarm whenever the limiting value is exceeded. This allows users to quickly know trends before individual samples have exceeded the set tolerances.
Upper CuSum curve: As a rule, however, there will be negative differences in addition to the positive ones. This means that the upper CuSum curve is moved back by the value exceeded, and the lower CuSum curve grows by the corresponding negative value. This idealized behavior makes these curves fluctuate more or less by the nominal value. In practice, however, we can observe that several measurements are in the same range as the tolerance band (i.e. either over or under the nominal value). This results in the possibility of a cumulative value that exceeds the defined limiting value and, therefore, generates an alarm. Also, small but continuous deviations can be made visible on one side since the differences are constantly cumulated.
Lower CuSum curve: Because it makes no sense to include all such small differences in the calculation, a range in which these differences are not included in the calculation can be defined. The limits that determine when an alarm is triggered can also be freely defined, of course, and are based on a pre-set, fixed process standard deviation. We suggest that you start with a mean variation value that is somewhat larger than the standard deviation of the period in question.
CuSum monitoring is not limited to a particular group of customers. With this tool, the user of this function is able to visually spot a trend or drift in his process before the individual measuring values exceed legal tolerances. However, the opportunity of regulating the process so that the positive differences do not dominate is just as important. In most cases, this means that more costs can be minimized.
Process capability
This function is concerned with the question of whether the process is at all capable of complying with the legal tolerances at this time. Unlike the functions described above, process capability calculates probability. This probability is also based on the Gaussian theory, and is continuously recalculated for all statistical periods in FreeWeigh.Net (hour, shift, day, week, month, year and batch). Mathematically, however, these calculations should be based on more than 25 samples, and the size of the samples should ideally include at least four individual values. In addition, the samples should be distributed over long periods of time so that the influencing factors of humans, machines, material, methods and environment can be changed as appropriate.
The parameters for the process capability index are the specification limits. This information determines the range within which the individual objects under consideration must be located. In the case of packaged products in the food industry, these are the values T2+ and T2-. The limits, however, can be defined more narrowly. Defining these limits establishes a tolerance within which the curve of the finite population must be located. To have a “quality-capable process”, the critical process capability (cpk) limit must be larger than 1.33. Values that are smaller than one are considered a “non-quality-capable process”, and values from 1 to 1.33 reflect a “conditionally quality-capable process”.
We basically distinguish between a process capability (cp) and a critical process capability (cpk). The reference number cp is a coded number on the expected percentage of errors in the process if the features are optimally distributed (i.e. symmetrically).
In practice, however, a symmetrical distribution of features is rare. This means that the distribution curve is either closer to the upper or lower specification limits. On the side on which the curve is closer to the specification limits, there is a greater potential for feature values that violate the limits. This critical mass is expressed by the reference number “critical process capability (cpk)”.
So, if cp = cpk, then the distribution is absolutely symmetrical. If the value of cpk is significantly smaller than that of cp, an attempt should be made to center the process better, if possible.
Process capability is especially useful for processes that demand a high degree of precision (small tolerances and small mean variations) and that use cost-intensive material. To interpret these indices and evaluate the quality of the process as a whole, specific experience and knowledge of the changeable influences and mean variations of the components is almost unavoidable.
This article was provided by Mettler Toledo. To learn more, visit http://us.mt.com.
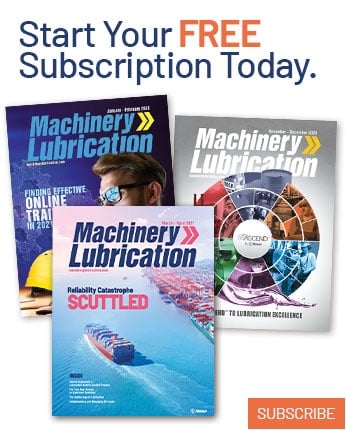