According to Wikipedia, machine learning “is the scientific study of algorithms and statistical models that computer systems use to perform a specific task effectively without using explicit instructions, relying on patterns and inference instead. It is seen as a subset of artificial intelligence.”
Machine learning is not a device you can plug into a production line and make the line operate better than before. It is a process that needs inputs from many devices to feed data to it so that data can be collected, evaluated and used to develop knowledge about how the production line works.
This knowledge can then be used to determine how the production line can have a higher throughput, operate at a lower cost and run more reliably. In this way, machine learning transforms an industrial operation into a system of systems that can get products to market faster at a lower cost so the company can remain competitive and keep its customers happy.
Process-based Machine Learning
Let’s add a modifier to the idea of machine learning and call it “process based.” This allows us to get to the heart of the matter in identifying the industrial technology that had to be created or modified because of the desire to use computer algorithms to enable the era of smart manufacturing.
Machine learning uses training data to teach its computer algorithm on what to expect from the production machines it’s monitoring to obtain that data.
It must rely on pattern recognition and inference to develop the capability for the algorithm to make decisions and predictions without having to write code to be explicitly programmed to perform that task. The training data is collected, processed and evaluated in a structured sequence of steps to prepare the data for use in the machine learning algorithm.
This structured sequence of steps is a process, and the creation of that process introduces new technologies in the form of devices to create the data, networks to store and process the data, and computers to process and clean the data for accuracy and relevancy.
That is a system represented by the image above. More importantly, that diagram is a system of systems (SoSe) due to the increasing complexity of its operation. We will see what that technology means to machine learning shortly.
Industrial Applications and Transformations Attributed to Machine Learning
The list of new technologies that can be attributed to machine learning is exhaustive and not able to be covered in its entirety within this article. Therefore, I will discuss the higher level issues that are more readily identifiable.
Predictive Maintenance
The possibility of predicting disruptions to a production line in advance can be invaluable to manufacturers. It allows the manager to schedule downtime at the most advantageous time and eliminate unscheduled downtime. Unscheduled downtime hits the profit margin hard and can result in the loss of your customer base. It also disrupts the supply chain, causing the carrying of excess stock.
The need to bring in additional manpower can cost a lot of money as well. A recent study predicted that the adoption of machine learning to enable predictive maintenance is expected to increase among manufacturers by 38 percent because of its ability to improve the profit margin by eliminating unscheduled work stoppages.
IT/OT Convergence and Network Security
The development of machine learning will also drive many business model modifications in a manufacturer’s standard operating procedures. This is especially true in the organizational makeup of a company. The computer network, which is the hallowed ground of the information technology (IT) department, must be co-located with the operational sensors on production machinery so data can be collected and sent to the data warehouse as training data for machine learning purposes.
There likely will be a need to tear down the wall of silence that separates the two groups internally to allow for collaboration and cooperation. After all, the floor operators and technicians will be significantly impacted if the network is not reliable or gets hacked, which can bring production to a stop. The operational technology (OT) sensors and devices will be affected as much as the IT network and computers.
Digital Twin Development
The ultimate objective of artificial intelligence and machine learning is to enable the development of a digital twin of the production floor. The creation of a digital twin should take place under a model-based systems engineering process using machine learning algorithms and knowledge gained as a foundation.
The digital twin can serve as a platform for running what-if scenarios to learn what we don’t know today. It can also be used as a model for designing higher reliability parts and adjusting the interactions between production-line machines to improve performance. The possibilities are endless.
About the Author
Joseph Zulick is a writer and manager at MRO Electric and Supply.
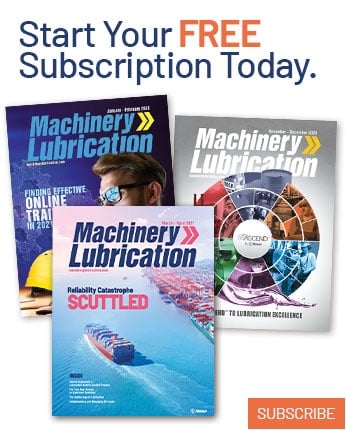