With fierce competition, uncontrollable economic factors and shrinking budgets, there is no room for inefficiency or error in today's global manufacturing industries. Gone are the days in which maintenance spare parts were stocked and purchased without cause or simply considered a necessary evil of doing business. Lean manufacturing and operational excellence now demands the utmost in efficiency, cost savings and performance at all times. Simply stated, companies must do more with less while increasing throughput to improve the bottom line.
One of the most common challenges for manufacturing and asset-intensive organizations lies within their material master data and asset management process. These particular companies often have multiple sites spread across large geographic regions, each with thousands of maintenance spare parts on hand to keep production running. In such large organizations, several different employees enter items into various enterprise systems, using different languages and little to no standard guidelines or communication.
Over time, this lack of standardization causes materials data to become inconsistent and unreliable, creating a significant barrier for maintenance and procurement, among a long list of other enterprise inefficiencies.
The most common effects of low-quality material master data include:
- Unidentifiable items
- Duplication
- Excess inventory accumulation
- False stock-outs
- Equipment downtime
- Inability to search and locate parts
- Increased maverick purchases (spot buys)
- Misleading and unreliable reporting
- Limited spend visibility
- Compromised enterprise system functionality
These major inefficiencies can cost companies a tremendous amount of time and money while preventing them from making critical business decisions. In the current era, where companies are forced to rely so heavily on business intelligence and technology, it isn't difficult to recognize the value of data quality and understand why data cleansing is quickly becoming a highly demanded service.
The Challenge
Through preventative and catastrophic repairs, material and vendor master data plays a critical role in the overall maintenance of facilities and operations. When every second of equipment downtime can cost a company thousands of dollars, it is absolutely crucial for materials and vendor data to be consistent, reliable and readily available at all times. Aside from maintenance, purchasing and procurement departments also rely heavily on master data for spend analysis, strategic sourcing and inventory control.
As manufacturing and asset-intensive organizations grow and evolve over time, so too does the volume of data housed within their enterprise system(s). Whether growth occurs by natural progression or via acquisitions and mergers, it adds a new degree of complexity to the already challenging master data management process. Through employee turnover, varying languages, multiple enterprise systems and little to no standard guidelines for data entry, master data progressively becomes inconsistent, inaccurate and saturated with duplication.
With potentially thousands of legacy records housed in the existing item master and new entries being made daily, improving data quality and changing traditional processes can be a laborious and time-consuming task. Add in the fact that most companies do not have the available resources, subject-matter expertise or tools to effectively perform data governance internally and the process becomes even more daunting.
The Solution
The ultimate objective and most efficient state for any organization is to operate on one common enterprise platform to enable visibility and communication across all business units. In order to accomplish this challenging task, legacy data must be merged together in preparation for migration into the chosen enterprise system.
While most companies are neglectfully aware of their data quality issues, it often isn't until legacy data is merged together that the severity of the situation becomes immediately and unavoidably evident. At this point, data cleansing is no longer an option but a necessity. The only remaining questions are who is going to cleanse it, how much is it going to cost and what standards are going to be used moving forward.
Data Cleansing
Data cleansing is defined as the process of analyzing, correcting and standardizing corrupt or inaccurate data records within a dataset. Despite some uneducated speculations, data cleansing is a niche service that requires specialized software, subject-matter expertise and a strategic project plan to manage an ever-changing dataset.
Without these three ingredients, companies that attempt to undertake a data-cleansing project internally are at risk of wasting significant time, money and resources, only to end up right where they began. The bottom line is that manufacturers specialize in manufacturing, not data cleansing. Therefore, outsourcing this task to a third-party service provider is often the most feasible and effective solution.
The data-cleansing process employs a comprehensive project plan, which combines automated software and manual effort to deliver the most useful master data. Whether cleansing is performed on material or vendor master data, the process typically involves a similar approach. In each project, data is tailored to the unique requirements of the enterprise system, company and industry in which they compete. Failure to do so may result in unmet expectations and mediocre data quality.
Data Evaluation and Needs Assessment
The first step in any data-cleansing project involves performing a detailed data evaluation to assess the item master's current state, establish a baseline for key performance indicators (KPIs) and determine project requirements. During the evaluation, a data analyst will perform a series of automated reports to assess the current condition of raw legacy data while selecting duplicate records and providing multiple before and after cleansing samples for review. The results of the data evaluation will be presented to the prospecting company in a formal report along with a detailed project proposal.
Establish Standard Operating Procedure
Upon proposal acceptance, the next step is to establish a corporate standard operating procedure, which will be enforced across the enterprise moving forward. The standard operating procedure should encompass all data components, including naming convention, abbreviations, classifications (product groups) and formatting requirements. During this stage, the service provider will offer expert consulting support as well as best-practice recommendations using an internally developed noun-modifier dictionary, abbreviation list and standard formatting templates.
The standard operating procedure and project plan should be customized and tailored specifically for the individual project. Establishing a clear and concise standard operating procedure that satisfies all business requirements at this time will provide the foundation for long-term data quality. Furthermore, the development of corporate standards should consider all variables and departmental influences, as this invaluable set of guidelines will soon become the internal law by which all future records are created and structured. Figure. 1 illustrates the noun-modifier-attribute naming convention methodology.
Figure 1. Noun-modifier dictionary sample
Clean, Standardize, Enhance and Validate
Once the standard operating procedure and project plan have been established, the cleansing process can begin. During the cleansing process, legacy data will pass through a series of automated software programs, which will identify, segregate and standardize the manufacturer name and part number. Next, using the pre-defined noun-modifier dictionary, each record will be assigned a noun-modifier pair as the primary and secondary identifier.
Based on the assigned noun-modifier pair, each record will also be assigned a corresponding list of attributes (characteristics), which will be populated with existing part information and standardized according to project guidelines. Any remaining attributes that have not yet been populated with legacy information will be researched and retrieved from pre-approved internal resources, manufacturer catalogs and online sources where available.
A test data sample containing 500 cleansed items should be provided approximately two weeks after project commencement to ensure the data standards and format are in compliance with business requirements.
A weekly conference call should be held between the service provider and project team to advise progress to date and address any questions or concerns relating to the data. Figure 2 outlines the various service level options available for data cleansing. In most projects, the objective is to clean data to the highest level possible, which includes attribute enhancement and classification. Alternatively, there are some projects in which business requirements or budgetary constraints require data to be cleansed to a less detailed level.
Figure 2. Data-cleansing service options
Quality Control Review
The quality control review process should be performed by a dedicated team of subject-matter experts who possess strong part knowledge and a high attention to detail. During this phase, each product group, manufacturer, part number and description is carefully reviewed and analyzed to ensure accuracy, completeness and compliance to project standards. A series of software programs further validate attribute information and check for any outstanding spelling mistakes or inconsistencies. Upon review and approval, each item is signed off and deemed "clean."
Duplicate and Review Items
Duplication represents one of the greatest inefficiencies within any given dataset, often accounting for as much as 20 percent of the item master. During the data-cleansing process, duplicates are identified by direct match and fit-form-function equivalent. Direct match duplicates are defined as items that possess the identical manufacturer name and part number.
Fit-form-function duplicates are defined as items that possess a different manufacturer name and/or part number yet are equivalent based on specifications including size, material and description. The itemized duplicate list is returned to the customer after cleansing, at which time the project team must advise a path forward for duplicate consolidation or removal.
In addition to duplication, 10 percent of a typical item master is generally found to be review items. Review items are defined as items lacking critical information for accurate part identification such as manufacturer name or part number. During the data-cleansing process, these items are flagged and compiled into a review list, which is then returned to the customer. A physical onsite review of the items in question is required to record the necessary part information for cleansing and inclusion in the item master.
Enterprise Formatting and File Delivery
Once the entire item master has been cleansed and approved by quality control, it is deemed complete and transferred to a team of information technology specialists. At this stage, software programs apply abbreviations according to project standards, and full descriptions are concatenated into short and long text fields as required in order to comply with enterprise system specifications. Proper data formatting and configuration at this stage will enable seamless uploading into the new enterprise system while ensuring maximum search and reporting functionality.
Once the data has been formatted to the enterprise system it is exported into a load-ready file and returned to the customer via electronic file transfer.
The Results
The aesthetic benefits of standardized, high-quality data are immediately evident following a data-cleansing initiative. The materials data now will have one consistent format and nomenclature across the enterprise, part descriptions will beam with attribute-rich descriptions, duplication will be nonexistent, and the data will be properly formatted to its respective fields.
More valuable than the aesthetic appearance is the extensive search and reporting functionality that has now been unlocked within the enterprise system. No longer are individual sites and departments operating blindly in silos or second-guessing every decision. With confidence, maintenance, purchasing and procurement can now perform efficient part searches, generate useful reports, conduct detailed spend analytics and identify idle inventory.
Using this increased level of business intelligence, the company can further improve efficiency and capture cost savings by disposing of excess/obsolete inventory, leveraging spend, optimizing inventory levels and enforcing compliance.
Key benefits of data cleansing include:
- Efficient part searchability
- Maintenance time savings
- Accurate reporting capabilities
- Identification and removal of duplicate items
- Excess inventory reduction
- Equipment downtime reduction
- Elimination of maverick purchases
- Maximum enterprise system functionality
Figure 3 displays a before-and-after data-cleansing sample, while Figure 4 outlines a duplicate example that has been identified across two different sites.
Figure 3. A before-and-after data-cleansing sample with attribute enhancement
Figure 4. A before-and-after duplicate sample with attribute enhancement
Data Governance
While data cleansing offers many immediate cost savings and efficiency gains, the data management challenge does not end once the initial project is complete. Maintaining high-quality data requires a rigorous data governance strategy to ensure ongoing accuracy, consistency and compliance throughout all future transactions. As new records are created and existing ones modified or suspended, it is critical that corporate standards and duplicate prevention be enforced across each and every data entry.
Various data governance solutions are available to provide a process that suits the unique aspects of each company. Web-based software solutions are becoming the most user-friendly and effective form of data governance. Each solution is strategically designed as a method of centralizing and streamlining the data governance process to maximize and maintain data quality while reducing administrative efforts and providing seamless system integration.
Implementing a Data-cleansing Initiative
Master data, which drives critical business decisions and daily operations, is arguably one of the most valuable assets that a company possesses. No matter how expensive, functionality-rich or industry-recommended an enterprise system may be, the harsh reality is that the software is only as good as the data flowing through it. Without the availability of high-quality master data, companies are essentially operating blind in a space that is complex and challenging. As economic factors and competition send markets into a vulnerable state, the push for cost savings and efficiency will become even more critical to the success and sustainability of business.
In an effort to achieve the highly sought after cost savings and efficiency gains that C-suite executives will inevitably demand, companies will turn to their enterprise systems and master data for the answers. Hence, implementing a data-cleansing initiative in parallel with an enterprise resource planning implementation may be one of the most valuable decisions a company can ever make. In the end, it will save a great deal of time, stress and money, in addition to the shame of numerous failed projects due to poor data.
About the Author
Jocelyn Facciotti is the marketing manager at IMA Ltd., a company specializing in maintenance, repair and operations (MRO) data cleansing and related services. For more information, visit www.imaltd.com.
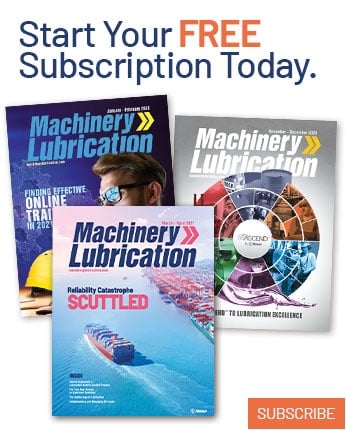